Data Observability vs Data Quality: Understanding the Differences and Importance
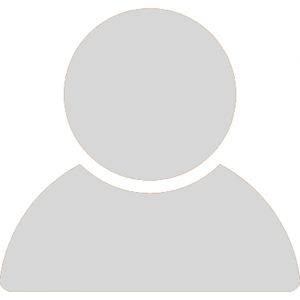
Audio : Listen to This Blog.
In today’s data-driven world, businesses heavily rely on data to make informed decisions, optimize operations, and drive growth. However, ensuring the reliability and usability of this data is not straightforward. Two crucial concepts that come into play here are data observability and data quality. Although they share some similarities, they serve different purposes and address distinct aspects of data management. This article delves into the differences and importance of data observability vs. data quality, highlighting how both practices work together to ensure data integrity and reliability, offering a comprehensive understanding of both.
Source: Cribl
What is Data Observability?
Source: acceldata
Data observability refers to the ability to fully understand and monitor the health and performance of data systems. It includes understanding data lineage, which helps track data flow, behavior, and characteristics. It involves monitoring and analyzing data flows, detecting anomalies, and gaining insights into the root causes of issues. Data observability provides a holistic view of the entire data ecosystem, enabling organizations to ensure their data pipelines function as expected.
Key Components of Data Observability
Source: TechTarget
Understanding the critical components of data observability is essential for grasping how it contributes to the overall health of data systems. These components enable organizations to gain deep insights into their data operations, identify issues swiftly, and ensure the continuous delivery of reliable data. Root cause analysis is a critical component of data observability, helping to identify the reasons behind inaccuracies, inconsistencies, and anomalies in data streams and processes. The following paragraphs explain each element in detail and highlight its significance.
Monitoring and Metrics in Data Pipelines
Monitoring and metrics form the backbone of data observability by continuously tracking the performance of data pipelines. Through real-time monitoring, organizations can measure various aspects such as throughput, latency, and error rates. These metrics provide valuable insights into the pipeline’s efficiency and identify bottlenecks or areas where performance may deteriorate.
Monitoring tools help set thresholds and generate alerts when metrics deviate from the norm, enabling proactive issue resolution before they escalate into significant problems. Data validation enforces predefined rules and constraints to guarantee data conforms to expectations, preventing downstream errors and ensuring data integrity.
Tracing
Tracing allows organizations to follow data elements through different data pipeline stages. By mapping the journey of data from its source to its destination, tracing helps pinpoint where issues occur and understand their impact on the overall process. Tracing is an integral part of data management processes, helping refine and improve how organizations manage their data.
For example, tracing can reveal whether the problem originated from a specific data source, transformation, or storage layer if data corruption is detected at a particular stage. This granular insight is invaluable for diagnosing problems and optimizing data workflows.
Logging
Logging captures detailed records of data processing activities, providing a rich source of information for troubleshooting and debugging. Logs document events, errors, transactions, and other relevant details within the data pipeline.
By analyzing logs, data engineers can identify patterns, trace the origins of issues, and understand the context in which they occurred. Effective logging practices ensure that all critical events are captured, making maintaining transparency and accountability in data operations easier. Data profiling involves analyzing datasets to uncover patterns, distributions, anomalies, and potential issues, aiding in effective data cleansing and ensuring data adheres to defined standards.
Alerting
Alerting involves setting up notifications to inform stakeholders when anomalies or deviations from expected behavior are detected in the data pipeline. Alerts can be configured based on predefined thresholds or anomaly detection algorithms. For instance, an alert could be triggered if data latency exceeds a specific limit or error rates spike unexpectedly.
Timely alerts enable rapid response to potential issues, minimizing their impact on downstream processes and ensuring that data consumers receive accurate and timely information. Alerting helps proactively identify and resolve data quality issues, ensuring accuracy, completeness, and consistency.
What is Data Quality?
Source: Alation
Data quality, on the other hand, focuses on the attributes that make data fit for its intended use. High-quality data is accurate, complete, consistent, timely, and relevant. Data quality involves processes and measures to cleanse, validate, and enrich data, making it reliable and valid for analysis and decision-making.
Data quality and observability are both crucial for ensuring data reliability and accuracy, focusing on real-time monitoring, proactive issue detection, and understanding data health and performance.
Key Dimensions of Data Quality
In data management, several key attributes determine the quality and effectiveness of data. Attributes such as accuracy, completeness, consistency, timeliness, and relevance ensure that data accurately reflects real-world entities, supports informed decision-making, and aligns with business objectives.
Accuracy
Accuracy is the degree to which data correctly represents the real-world entities it describes. Inaccurate data can lead to erroneous conclusions and misguided business decisions. Ensuring accuracy involves rigorous validation processes that compare data against known standards or sources of truth.
For example, verifying customer addresses against official postal data can help maintain accurate records. High accuracy enhances the credibility of data and ensures that analyses and reports based on this data are reliable.
Completeness
Completeness refers to the extent to which all required data is available, and none is missing. Incomplete data can obscure critical insights and lead to gaps in analysis. Organizations must implement data collection practices that ensure all necessary fields are populated, and no vital information is overlooked.
For instance, ensuring that all customer profiles contain mandatory details like contact information and purchase history is essential for comprehensive analysis. Complete data sets enable more thorough and meaningful interpretations.
Consistency
Consistency ensures uniformity of data across different datasets and systems. Inconsistent data can arise from discrepancies in data formats, definitions, or values used across various sources. Standardizing data entry protocols and implementing data integration solutions can help maintain consistency.
For example, using a centralized data dictionary to define key terms and formats ensures that all departments interpret data uniformly. Consistent data enhances comparability and reduces misunderstandings.
Timeliness
Timeliness means that data is up-to-date and available when needed. Outdated data can lead to missed opportunities and incorrect assessments. Organizations should establish processes for regular data updates and synchronization to ensure timeliness.
For instance, real-time data feeds from transaction systems can keep financial dashboards current. Timely data enables prompt decision-making and responsiveness to changing circumstances.
Relevance
Relevance ensures that data is pertinent to the context and purpose for which it is used. Irrelevant data can clutter analysis and dilute focus. Organizations must align data collection and maintenance efforts with specific business objectives to ensure relevance.
For example, collecting data on user interactions with a website can inform targeted marketing strategies. Relevant data supports precise and actionable insights, enhancing the value derived from data analysis.
Data Observability vs. Data Quality: Key Differences
Source: DQOps
Quality and data observability safeguard data-driven decisions, maintain data integrity, and address real-time issues. Here is a list of the key differences between the two:
1. Scope
The scope of data observability focuses on monitoring and understanding the data ecosystem’s health and performance. It encompasses the entire data pipeline, from ingestion to delivery, and ensures that all components function cohesively.
Data quality, however, is concerned with the intrinsic attributes of the data itself, aiming to enhance its fitness for purpose. While observability tracks the operational state of data systems, quality measures assess the data’s suitability for analysis and decision-making.
2. Approach
The approach to achieving data observability involves monitoring, tracing, logging, and alerting. These methods provide real-time visibility into data processes, enabling quick identification and resolution of issues. Data quality enhances data attributes using cleansing, validation, and enrichment processes.
It involves applying rules and standards to improve data accuracy, completeness, consistency, timeliness, and relevance. While observability ensures smooth data flow, quality management ensures the data is valuable and trustworthy. Implementing data quality and observability practices involves systematic and strategic steps, including data profiling, cleansing, validation, and observability.
3. Goals
The primary goal of data observability is to ensure the smooth functioning of data pipelines and early detection of problems. Organizations can prevent disruptions and maintain operational efficiency by maintaining robust observability practices. In contrast, data quality aims to provide accurate, complete, consistent, timely, and relevant data for analysis and decision-making.
High-quality data supports reliable analytics, leading to more informed business strategies. Both observability and quality are essential for a holistic data management strategy, but they focus on different objectives.
Why Both Matter
Understanding the differences between data observability and data quality highlights why both are crucial for a robust data strategy. Organizations need comprehensive visibility into their data systems to maintain operational efficiency and quickly address issues. Simultaneously, they must ensure their data meets quality standards to support reliable analytics and decision-making.
Benefits of Data Observability
Source: InTechHouse
High-quality data is essential for deriving precise business intelligence, making informed decisions, and maintaining regulatory compliance. Organizations can unlock valuable insights, support better decision-making, and meet industry standards by ensuring data accuracy.
Accurate Insights: High-quality data leads to more precise and actionable business intelligence. Accurate data forms the foundation of reliable analytics and reporting, enabling organizations to derive meaningful insights from their data.
With accurate insights, businesses can more precisely identify trends, spot opportunities, and address challenges, leading to more effective strategies and improved outcomes.
Better Decision-Making: Reliable data supports informed and effective strategic decisions. When decision-makers have access to high-quality data, they can base their choices on solid evidence rather than assumptions.
This leads to better-aligned strategies, optimized resource allocation, and improved overall performance. Reliable data empowers organizations to navigate complex environments confidently and make decisions that drive success.
Regulatory Compliance: Adhering to data quality standards helps meet regulatory requirements and avoid penalties. Many industries have strict data regulations that mandate accurate and reliable data handling.
Organizations can ensure compliance with these regulations by maintaining high data quality and reducing the risk of legal and financial repercussions. Regulatory compliance enhances the organization’s reputation and builds trust with customers and partners.
Conclusion
In the debate of data observability vs data quality, it is clear that both play vital roles in ensuring the effectiveness of an organization’s data strategy. While data observability provides the tools to monitor and maintain healthy data systems, data quality ensures the data is reliable and valuable. By integrating both practices, organizations can achieve a comprehensive approach to managing their data, ultimately leading to better outcomes and sustained growth.
Do you have any further questions or need additional insights on this topic?