Prescriptive Analytics: Definition, Tools, and Techniques for Better Decision Making
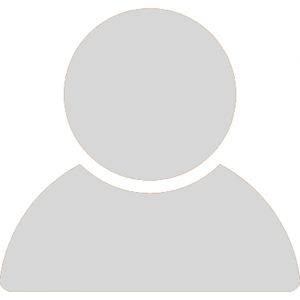
Audio : Listen to This Blog.
In today’s data-driven world, businesses constantly seek ways to enhance their decision-making processes. Understanding how prescriptive analytics works is crucial; it involves analyzing data to provide specific recommendations that improve business outcomes and support decision-making. Prescriptive analytics stands out as a powerful tool, helping organizations not only understand what has happened and why but also providing recommendations on what should be done next. This blog will delve into prescriptive analytics, exploring its definition, tools, techniques, and how it can be leveraged for better decision-making in 2024.
What is Prescriptive Analytics?
Prescriptive analytics is the third phase of business analytics, following descriptive and predictive analytics. While descriptive analytics focuses on what happened and predictive analytics forecasts what might happen, prescriptive analytics goes a step further. It uses current and historical data to make recommendations. It suggests actions to take for optimal outcomes based on the data.
Key Characteristics of Prescriptive Analytics:
- Action-Oriented: Unlike other forms of analytics, prescriptive analytics provides actionable recommendations.
- Optimization-Focused: It aims to find the best possible solution or decision among various alternatives.
- Utilizes Predictive Models: It often incorporates predictive analytics to forecast outcomes and then recommends actions based on those predictions.
- Incorporates Business Rules: It considers organizational rules, constraints, and goals to provide feasible solutions.
- Improves Decision-Making: Prescriptive analytics techniques improve decision-making by suggesting the best possible business outcomes.
- Synthesizes Insights: Prescriptive analytics work by synthesizing insights from descriptive, diagnostic, and predictive analytics, using advanced algorithms and machine learning to answer the question ‘What should we do about it?’
Prescriptive Analytics Software Tools
Several tools are available to help businesses implement prescriptive analytics. Scalability is crucial in prescriptive analytics software, especially in handling increasing data loads as businesses grow, such as during sale seasons for ecommerce companies. These tools range from software solutions to more complex platforms, offering a variety of functionalities. Here are some notable prescriptive analytics tools:
1. IBM Decision Optimization
IBM Decision Optimization uses advanced algorithms and machine learning to provide precise recommendations. It integrates well with IBM’s data science products, making it a robust tool for large enterprises.
2. Google Cloud AI
Google Cloud AI offers tools for building and deploying machine learning models, and its optimization solutions can help businesses make data-driven decisions. Google’s AI platform is known for its scalability and reliability.
3. Microsoft Azure Machine Learning
Azure’s machine learning suite includes prescriptive analytics capabilities. It provides a comprehensive environment for data preparation, model training, and deployment, and integrates seamlessly with other Azure services.
4. SAP Analytics Cloud
SAP Analytics Cloud combines business intelligence, predictive analytics, and planning capabilities in one platform. Its prescriptive analytics tools are designed to help businesses make well-informed decisions.
5. TIBCO Spotfire
TIBCO Spotfire is an analytics platform that offers prescriptive analytics features. It supports advanced data visualization, predictive analytics, and integrates with various data sources.
Techniques in Prescriptive Analytics
Prescriptive analytics involves various techniques to derive actionable insights from data. These techniques are used to analyze data and provide recommendations on the optimal course of action or strategy moving forward. Prescriptive analytics also involves the analysis of raw data about past trends and performance to determine possible courses of action or new strategies. Here are some key techniques:
1. Optimization Algorithms
Optimization algorithms are at the heart of prescriptive analytics. They help find the best possible solution for a given problem by considering constraints and objectives. Common optimization algorithms include:
- Linear Programming: Solves problems with linear constraints and objectives.
- Integer Programming: Similar to linear programming but involves integer variables.
- Nonlinear Programming: Deals with problems where the objective or constraints are nonlinear.
2. Simulation
Simulation involves creating a model of a real-world process and experimenting with different scenarios to see their outcomes. This technique helps in understanding the potential impact of different decisions.
3. Heuristics
Heuristics are rule-of-thumb strategies used to make decisions quickly when an exhaustive search is impractical. They provide good enough solutions that are found in a reasonable time frame.
4. Machine Learning
Machine learning models, particularly those that predict future outcomes, play a crucial role in prescriptive analytics. These models help forecast scenarios, which are then used to recommend actions. Data analytics is essential in this process, as it involves using machine learning to process quality data for accurate prescriptive analytics.
5. Monte Carlo Simulation
Monte Carlo simulation is a technique that uses randomness to solve problems that might be deterministic in principle. It’s used to model the probability of different outcomes in a process that cannot easily be predicted.
Applications of Prescriptive Analytics in 2024
Prescriptive analytics can be applied across various industries to enhance decision-making processes. By simulating a range of approaches to a given business problem, prescriptive analytics can determine future performance based on interdependencies and modeling the entire business. It is important to understand the relationship between predictive and prescriptive analytics; while predictive analytics forecasts future trends and outcomes based on historical data, prescriptive analytics offers actionable recommendations and specific steps for achieving desired outcomes. Here are some examples:
1. Supply Chain Management
Prescriptive analytics helps optimize supply chain operations by recommending actions to reduce costs, improve efficiency, and ensure timely delivery. It can suggest the best routes for transportation, optimal inventory levels, and efficient production schedules.
2. Healthcare
In healthcare, prescriptive analytics can recommend treatment plans for patients, optimize resource allocation, and improve operational efficiency. It can also help in managing patient flow and reducing waiting times in hospitals.
3. Finance
Financial institutions use prescriptive analytics to manage risk, optimize investment portfolios, and detect fraudulent activities. It can recommend strategies for maximizing returns while minimizing risk.
4. Retail
Retailers leverage prescriptive analytics to optimize pricing strategies, manage inventory, and enhance customer experience. It can suggest personalized product recommendations and promotional offers.
5. Manufacturing
In manufacturing, prescriptive analytics can optimize production schedules, reduce downtime, and improve quality control. It can recommend maintenance schedules to prevent equipment failure and minimize disruptions.
Challenges in Implementing Prescriptive Analytics
Despite its benefits, implementing prescriptive analytics comes with challenges. Historical data is crucial in prescriptive analytics as it helps make accurate predictions and offers specific recommendations for strategic decisions. Additionally, diagnostic analytics plays a vital role in understanding data by delving into the root causes of past events, which enhances the depth of insights for prescriptive analytics.
1. Historical Data Quality and Integration
High-quality data is crucial for effective prescriptive analytics. Organizations often struggle with data silos and inconsistencies, making it challenging to integrate and prepare data for analysis.
2. Complexity
Prescriptive analytics involves complex algorithms and models, requiring specialized skills to implement and interpret. Organizations may face difficulties in finding and retaining skilled professionals.
3. Scalability
Scaling prescriptive analytics solutions to handle large datasets and complex problems can be challenging. It requires robust infrastructure and computational power.
4. Cost
Implementing prescriptive analytics solutions can be costly. Organizations need to invest in technology, infrastructure, and skilled personnel.
5. Change Management
Adopting prescriptive analytics requires a cultural shift within the organization. Employees need to trust and rely on data-driven recommendations, which can be a significant change from traditional decision-making processes.
The Future of Prescriptive Analytics
As we move into 2024, several trends are shaping the future of prescriptive analytics:
1. Explainable AI (XAI)
Explainable AI is becoming increasingly important as organizations seek transparency in their decision-making processes. XAI helps build trust by making it easier to understand how and why specific recommendations are made.
2. Integration with IoT
The Internet of Things (IoT) generates vast amounts of data that can be used in prescriptive analytics. Integrating IoT data can provide real-time insights and enhance decision-making processes.
3. Cloud Computing
Cloud computing is making prescriptive analytics more accessible by providing scalable infrastructure and tools. It allows organizations to process and analyze large datasets without significant upfront investment in hardware.
4. AI and Machine Learning Advances
Advances in AI and machine learning are continuously improving the capabilities of prescriptive analytics. New algorithms and models are making it possible to solve more complex problems and provide more accurate recommendations.
5. Ethical Considerations
As the use of prescriptive analytics grows, so do concerns about ethics and fairness. Organizations must ensure their analytics processes are transparent, unbiased, and respect privacy.
Wrapping Up
Prescriptive analytics is a powerful tool that helps businesses make better decisions by providing actionable recommendations. By leveraging tools like IBM Decision Optimization, Google Cloud AI, Microsoft Azure Machine Learning, SAP Analytics Cloud, and TIBCO Spotfire, organizations can harness the power of prescriptive analytics to optimize operations, enhance efficiency, and drive growth.
However, implementing prescriptive analytics comes with challenges, including data quality, complexity, scalability, cost, and change management. As we move into 2024, trends like explainable AI, IoT integration, cloud computing, advances in AI, and ethical considerations will shape the future of prescriptive analytics.
By embracing these trends and overcoming challenges, businesses can fully realize the potential of prescriptive analytics and make smarter, data-driven decisions.
For more insights on Analytics and its applications, read our blogs:
AI in Predictive Analytics Solutions: Unlocking Future Trends and Patters in the USA (2024 & Beyond)
Predictive Analytics Solutions for Business Growth in Georgia