Top Predictive Analytics Tools in 2024
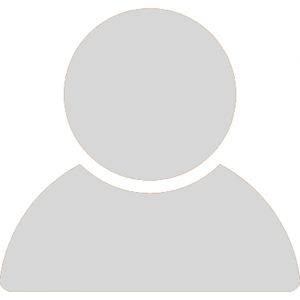
Audio : Listen to This Blog.
Predictive analytics has revolutionized how businesses make decisions, enabling them to leverage data to forecast trends, optimize operations, and enhance customer experiences. Predictive analysis tools play a crucial role in this process by utilizing statistics, data science, machine learning, and artificial intelligence techniques to improve business functions and predict future events. As we navigate through 2024, the tools available for predictive analytics are more advanced, user-friendly, and powerful than ever. This blog explores the top predictive analytics tools of 2024 that are transforming data-driven decision-making for businesses of all sizes.
Understanding Predictive Analytics
Predictive analytics involves using historical data, statistical algorithms, and machine learning techniques to predict future outcomes. By leveraging predictive analytics capabilities, businesses can make informed decisions, mitigate risks, and uncover opportunities. The primary benefits of predictive analytics include:
- Better Decision-Making: Provides insights that guide strategic planning.
- Efficiency Improvement: Optimizes business processes to reduce waste.
- Customer Experience Enhancement: Anticipates customer needs and behaviors.
- Risk Management: Predicts and mitigates potential risks.
- Innovation: Identifies new market opportunities and trends.
What are Predictive Analytics Tools?
Predictive analytics tools are software applications that leverage statistical modeling, machine learning, and data mining techniques to identify patterns and relationships within historical data. These tools often include predictive analytics features such as data visualizations, reports, and dashboards. These patterns are then used to make predictions about future events or outcomes.
Benefits of Using Predictive Analytics Tools:
- Competitive Advantage: In today’s data-driven world, businesses that leverage predictive analytics gain a significant edge over competitors. They can make quicker, more informed decisions, identify market opportunities faster, and optimize their operations for maximum efficiency. Predictive analytics models, such as regression, classification, and neural networks, contribute to better decision-making by simplifying development, feature engineering, and model selection.
- Increased Revenue: Predictive analytics can help businesses optimize pricing strategies, personalize marketing efforts, and identify new sales opportunities.
- Reduced Costs: By proactively identifying potential issues, businesses can take steps to prevent them, leading to cost savings.
- Boost Innovation: By uncovering hidden patterns and trends, predictive analytics can spark new ideas and lead to innovative products and services.
- Improve Operational Efficiency: By streamlining processes and optimizing resource allocation, predictive analytics can help businesses operate more efficiently and productively.
Top Predictive Analytics Tools in 2024
The landscape of predictive analytics platforms is constantly evolving. Here are some of the top contenders in 2024, catering to different needs and budgets:
1. IBM Watson Studio
Overview: IBM Watson Studio is a leading data science and machine learning platform that allows businesses to build, train, and deploy models at scale. It integrates various tools and technologies to facilitate comprehensive data analysis. IBM Watson Studio also enhances the development and deployment of predictive models, making it easier for businesses to create responsible and explainable predictive analytics.
Key Features:
- Automated Data Preparation: Streamlines the data cleaning and preparation process.
- AI Model Lifecycle Management: Supports the entire lifecycle of AI models from development to deployment.
- Integration with Open Source Tools: Compatible with Python, R, and Jupyter notebooks.
- Collaboration: Enhances teamwork with shared projects and workflows.
Use Cases:
- Healthcare: Predicting patient outcomes.
- Finance: Fraud detection and risk assessment.
- Retail: Demand forecasting and inventory management.
2. SAS Predictive Analytics
Overview: SAS provides a robust suite of predictive analytics tools known for their advanced data mining, machine learning, and statistical analysis capabilities. SAS supports the development and optimization of analytics models, including predictive modeling, feature engineering, and model selection.
Key Features:
- Advanced Analytics: Offers powerful statistical and machine learning techniques.
- Data Visualization: Intuitive visualizations to easily interpret data.
- Real-Time Analytics: Enables real-time data analysis and predictions.
- Scalability: Efficiently handles large datasets.
Use Cases:
- Marketing: Personalized marketing and customer segmentation.
- Manufacturing: Predictive maintenance and quality control.
- Telecommunications: Customer churn prediction and network optimization.
3. Google Cloud AI Platform
Overview: Google Cloud AI Platform provides a comprehensive suite of machine learning tools that allow developers and data scientists to build, train, and deploy models on Google’s cloud infrastructure. Additionally, it supports the entire machine learning workflow with its robust predictive analytics software, which integrates ML and AI to enhance predictive focus and data sourcing.
Key Features:
- End-to-End ML Pipeline: Supports the entire machine learning workflow.
- AutoML: Enables non-experts to create high-quality machine learning models.
- Scalability: Utilizes Google’s robust cloud infrastructure.
- BigQuery Integration: Seamlessly integrates with Google’s data warehouse for large-scale data analysis.
Use Cases:
- Retail: Personalizing shopping experiences and improving customer retention.
- Finance: Risk management and fraud detection.
- Healthcare: Enhancing diagnostic accuracy and treatment plans.
4. Microsoft Azure Machine Learning
Overview: Microsoft Azure Machine Learning is a cloud-based environment designed for building, training, and deploying machine learning models. It supports the entire lifecycle of predictive analytics, making it a comprehensive predictive analytics solution.
Key Features:
- Automated Machine Learning: Simplifies model building and deployment.
- ML Ops: Facilitates the operationalization and management of models.
- Integration with Azure Services: Deep integration with other Microsoft Azure services.
- Interactive Workspaces: Collaborative environment for data scientists and developers.
Use Cases:
- Finance: Credit scoring and risk assessment.
- Retail: Sales forecasting and inventory optimization.
- Manufacturing: Predictive maintenance and production optimization.
5. Tableau
Overview: Tableau is a leading data visualization tool that also offers advanced analytics capabilities, making it a powerful platform for predictive analytics. As a comprehensive data analytics platform, Tableau supports advanced analytics and data visualization, enabling users to execute complex data processing tasks with ease.
Key Features:
- Interactive Dashboards: User-friendly dashboards for data exploration.
- Integration with R and Python: Supports advanced analytics with integration to popular programming languages.
- Real-Time Data Analysis: Processes and analyzes data in real-time.
- Visual Analytics: Strong focus on creating intuitive visualizations for better data insights.
Use Cases:
- Sales: Performance analysis and forecasting.
- Marketing: Customer segmentation and targeting.
- Finance: Financial forecasting and analysis.
6. RapidMiner
Overview: RapidMiner is an open-source data science platform that provides a range of tools for data preparation, machine learning, and model deployment. It supports the entire data science workflow with robust predictive analytics capabilities.
Key Features:
- Visual Workflow Designer: Intuitive drag-and-drop interface for creating workflows.
- Automated Machine Learning: Facilitates the creation of machine learning models with minimal manual intervention.
- Scalability: Efficiently handles large datasets and complex workflows.
- Big Data Integration: Supports integration with Hadoop and Spark for big data analytics.
Use Cases:
- Retail: Customer behavior prediction and segmentation.
- Telecommunications: Network optimization and customer churn prediction.
- Healthcare: Predictive diagnostics and patient management.
7. H2O.ai
Overview: H2O.ai offers an open-source machine learning platform known for its speed and scalability, providing tools for building, training, and deploying machine learning models. The platform supports the development and deployment of various predictive analytics models, including regression, classification, time series, clustering, neural network, decision trees, and ensemble models.
Key Features:
- AutoML: Automates the process of building machine learning models.
- Scalability: Efficiently handles large-scale data processing.
- Integration with R and Python: Supports integration with popular programming languages for advanced analytics.
- Visualization Tools: Provides robust tools for creating intuitive data visualizations.
Use Cases:
- Finance: Predictive modeling for investment strategies and risk assessment.
- Healthcare: Predicting patient outcomes and improving treatment plans.
- Insurance: Risk assessment and fraud detection.
8. TIBCO Statistica
Overview: TIBCO Statistica is an advanced analytics platform offering a comprehensive suite of tools for data analysis, machine learning, and data visualization. It integrates seamlessly with other analytics tools, including SAP Analytics Cloud, to enhance predictive analytics, data visualizations, and business insights.
Key Features:
- Data Preparation: Powerful tools for data cleaning and preparation.
- Machine Learning: Supports a wide range of machine learning algorithms.
- Real-Time Analytics: Enables real-time data processing and analysis.
- Integration: Seamless integration with other TIBCO analytics tools.
Use Cases:
- Manufacturing: Predictive maintenance and quality control.
- Healthcare: Patient risk stratification and management.
- Retail: Customer behavior analysis and demand forecasting.
Conclusion
In 2024, predictive analytics tools are more advanced and accessible than ever before, enabling businesses to harness the power of their data for strategic decision-making. By leveraging these tools, organizations can improve efficiency, enhance customer experiences, mitigate risks, and drive innovation.
Each tool listed here offers unique strengths and features, making it essential to choose the one that best fits your organization’s specific needs and goals. Whether you’re looking to optimize operations, predict customer behavior, or uncover new business opportunities, there is a predictive analytics tool tailored to your needs.
For more insights on Predictive Analytics and its applications, read our blogs: